A ‘fair-trade data’ standard for AI
In recent months, policymakers have begun turning their attention to the potential hazards and perils that may come as we turn over an increasing amount of decision-making responsibility to artificial intelligence or “AI.” These are not the Hollywood-driven fears of AI-gone-mad that found their way into our cultural subconscious through films and TV; rather, the risk posed by AI as it exists today is far subtler and arguably more insidious. The threat is not a hostile takeover by a malevolent computer, but instead the “baking in” of human prejudices, biases and injustices into seemingly dispassionate computer code.
AI and machine learning systems only work if they’re given a stream of data — data collected from countless individuals that is fed into a series of algorithms and used to help streamline complex decisions. But these decisions have real, tangible consequences: Does a mortgage get approved? Will insurance cover a medical procedure? Which resumes are surfaced to hiring managers?
{mosads}It’s easy to see how the wrong data or algorithm could inadvertently replicate conscious or unconscious human biases and bake them into systems too complex and deeply embedded for anyone to reasonably monitor. And so, as members of Congress and other policymakers look to build a framework for the ethical development and deployment of artificial intelligence, the key thing they must consider is where data is coming from, how it’s being processed, and if it respects the privacy and anonymity of the individuals who supply it.
In the 1990s, the term “conflict resource” entered the public consciousness — precious, valuable materials being mined and sold for the purpose of funding violence and exploitation. As part of a global outcry against these materials, an infrastructure was created to certify “conflict-free” or “fair trade” supply chains, allowing for more ethical use and consumption of necessary materials. As data becomes the currency of an AI-driven economy, we must similarly build an infrastructure that limits and sanctions “conflict data” and promotes an ethical “fair trade data” standard.
So, what would “fair trade data” look like?
First, it must be subject to technically enforced data use minimization control — this is to say that data should have embedded controls so it can only be used for authorized intended purposes. Demographic data collected to identify potential medical conditions, for instance, should not find itself used to better target advertisements.
Second, it must be designed to decrease bias and discrimination as much as possible. This is made possible by dynamic functional separation of data sets. This means that individual data sets — names, birthdates, addresses, gender, etc. — are kept separate in all instances, except when they need to be relinked for a specific purpose, based on authorized use and established regulations. If a class like race or gender does not need to apply to an AI task, it should not be considered, even if that data is available.
Last, it must only be stored and shared with built-in safeguards like dynamic use-case specific de-identification designed to preserve the privacy rights of individuals to ensure that it stays “ethical” even if the data set is leaked or as regulations evolve.
{mossecondads}With these principles at its core, “fair trade data” is designed to both maintain fidelity of the information and reduce the possibility of re-identification, bias and discrimination. At the same time, it still allows for researchers, companies, government agencies and more to use data, ethically, to solve big problems, streamline complex processes, and make difficult decisions. If these kinds of protections are not put into place, individuals will suffer as their data is processed in unforeseen ways, shared with unethical actors or used to reinforce structural and systemic biases.
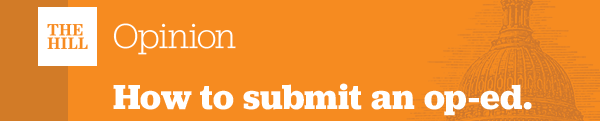
This is the greatest challenge for policymakers — regulating the future of data and AI means making rules for a world we do not yet fully understand. AI is in its infancy, but that means the time is now to put safeguards in place to ensure that individuals are protected while still fostering an environment that encourages innovation. By setting standards for data usage, storage and sharing, we drastically reduce the chances that an algorithm is able to cause unintentional harm to any group or individual.
Gary LaFever is CEO and General Counsel of Anonos, a technology firm specializing in data risk management, security and privacy. LaFever has been consulted by leading global corporations, international regulatory bodies and the United States Congress for his expertise on data privacy. He was formerly a partner at the top-rated international law firm of Hogan Lovells. Follow him on Twitter @GaryLaFever and on LinkedIn.
Copyright 2024 Nexstar Media Inc. All rights reserved. This material may not be published, broadcast, rewritten, or redistributed..