Will Artificial Intel get along with us? Only if we design it that way

Artificial Intelligence (AI) systems that interact with us the way we interact with each other have long typified Hollywood’s image, whether you think of HAL in “2001: A Space Odyssey,” Samantha in “Her,” or Ava in “Ex Machina.” It thus might surprise people that making systems that interact, assist or collaborate with humans has never been high on the technical agenda.
From its beginning, AI has had a rather ambivalent relationship with humans. The biggest AI successes have come either at a distance from humans (think of the “Spirit” and “Opportunity” rovers navigating the Martian landscape) or in cold adversarial faceoffs (the Deep Blue defeating world chess champion Gary Kasparov, or AlphaGo besting Lee Sedol). In contrast to the magnetic pull of these “replace/defeat humans” ventures, the goal of designing AI systems that are human-aware, capable of interacting and collaborating with humans and engendering trust in them, has received much less attention.
More recently, as AI technologies started capturing our imaginations, there has been a conspicuous change — with “human” becoming the desirable adjective for AI systems. There are so many variations — human-centered, human-compatible, human-aware AI, etc. — that there is almost a need for a dictionary of terms. Some of this interest arose naturally from a desire to understand and regulate the impacts of AI technologies on people. In previous columns, I’ve looked, for example, at bias in AI systems and the impact of AI-generated synthetic reality, such as deep fakes or “mind twins.”
This time, let us focus on the challenges and impacts of AI systems that continually interact with humans — as decision support systems, personal assistants, intelligent tutoring systems, robot helpers, social robots, AI conversational companions, etc.
To be aware of humans, and to interact with them fluently, an AI agent needs to exhibit social intelligence. Designing agents with social intelligence received little attention when AI development was focused on autonomy rather than coexistence. Its importance for humans cannot be overstated, however. After all, evolutionary theory shows that we developed our impressive brains not so much to run away from lions on the savanna but to get along with each other.
A cornerstone of social intelligence is the so-called “theory of mind” — the ability to model mental states of humans we interact with. Developmental psychologists have shown (with compelling experiments like the Sally-Anne test) that children, with the possible exception of those on the autism spectrum, develop this ability quite early.
Successful AI agents need to acquire, maintain and use such mental models to modulate their own actions. At a minimum, AI agents need approximations of humans’ task and goal models, as well as the human’s model of the AI agent’s task and goal models. The former will guide the agent to anticipate and manage the needs, desires and attention of humans in the loop (think of the prescient abilities of the character Radar on the TV series “M*A*S*H*”), and the latter allow it to act in ways that are interpretable to humans — by conforming to their mental models of it — and be ready to provide customized explanations when needed.
With the increasing use of AI-based decision support systems in many high-stakes areas, including health and criminal justice, the need for AI systems exhibiting interpretable or explainable behavior to humans has become quite critical. The European Union’s General Data Protection Regulation posits a right to contestable explanations for all machine decisions that affect humans (e.g., automated approval or denial of loan applications). While the simplest form of such explanations could well be a trace of the reasoning steps that lead to the decision, things get complex quickly once we recognize that an explanation is not a soliloquy and that the comprehensibility of an explanation depends crucially on the mental states of the receiver. After all, your physician gives one kind of explanation for her diagnosis to you and another, perhaps more technical one, to her colleagues.
Provision of explanations thus requires a shared vocabulary between AI systems and humans, and the ability to customize the explanation to the mental models of humans. This task becomes particularly challenging since many modern data-based decision-making systems develop their own internal representations that may not be directly translatable to human vocabulary. Some emerging methods for facilitating comprehensible explanations include explicitly having the machine learn to translate explanations based on its internal representations to an agreed-upon vocabulary.
AI systems interacting with humans will need to understand and leverage insights from human factors and psychology. Not doing so could lead to egregious miscalculations. Initial versions of Tesla’s auto-pilot self-driving assistant, for example, seemed to have been designed with the unrealistic expectation that human drivers can come back to full alertness and manually override when the self-driving system runs into unforeseen modes, leading to catastrophic failures. Similarly, the systems will need to provide an appropriate emotional response when interacting with humans (even though there is no evidence, as yet, that emotions improve an AI agent’s solitary performance). Multiple studies show that people do better at a task when computer interfaces show appropriate affect. Some have even hypothesized that part of the reason for the failure of Clippy, the old Microsoft Office assistant, was because it had a permanent smug smile when it appeared to help flustered users.
AI systems with social intelligence capabilities also produce their own set of ethical quandaries. After all, trust can be weaponized in far more insidious ways than a rampaging robot. The potential for manipulation is further amplified by our own very human tendency to anthropomorphize anything that shows even remotely human-like behavior. Joe Weizenbaum had to shut down Eliza, history’s first chatbot, when he found his staff pouring their hearts out to it; and scholars like Sherry Turkle continue to worry about the artificial intimacy such artifacts might engender. Ability to manipulate mental models can also allow AI agents to engage in lying or deception with humans, leading to a form of “head fakes” that will make today’s deep fakes tame by comparison. While a certain level of “white lies” are seen as the glue for human social fabric, it is not clear whether we want AI agents to engage in them.
As AI systems increasingly become human-aware, even quotidian tools surrounding us will start gaining mental-modeling capabilities. This adaptivity can be both a boon and a bane. While we talked about the harms of our tendency to anthropomorphize AI artifacts that are not human-aware, equally insidious are the harms that can arise when we fail to recognize that what we see as a simple tool is actually mental-modeling us. Indeed, micro-targeting by social media can be understood as a weaponized version of such manipulation; people would be much more guarded with social media platforms if they realized that those platforms are actively profiling them.
Given the potential for misuse, we should aim to design AI systems that must understand human values, mental models and emotions, and yet not exploit them with intent to cause harm. In other words, they must be designed with an overarching goal of beneficence to us.
All this requires a meaningful collaboration between AI and humanities — including sociology, anthropology and behavioral psychology. Such interdisciplinary collaborations were the norm rather than the exception at the beginning of the AI field and are coming back into vogue.
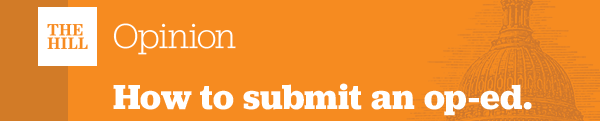
Formidable as this endeavor might be, it is worth pursuing. We should be proactively building a future where AI agents work along with us, rather than passively fretting about a dystopian one where they are indifferent or adversarial. By designing AI agents to be human-aware from the ground up, we can increase the chances of a future where such agents both collaborate and get along with us.
Subbarao Kambhampati, PhD, is a professor of computer science at Arizona State University and the Chief AI Officer for AI Foundation, which develops realistic AI companions with social skills. He was the president of the Association for the Advancement of Artificial Intelligence, a founding board member of Partnership on AI, and is an Innovators Network Foundation Privacy Fellow. He can be followed on Twitter @rao2z.
Copyright 2024 Nexstar Media Inc. All rights reserved. This material may not be published, broadcast, rewritten, or redistributed..